🕗 4 min read ・An inside view of Data Science's role at the core of relevanC's personalised promotions platform, Lead Data Scientist, Robin Monnier.
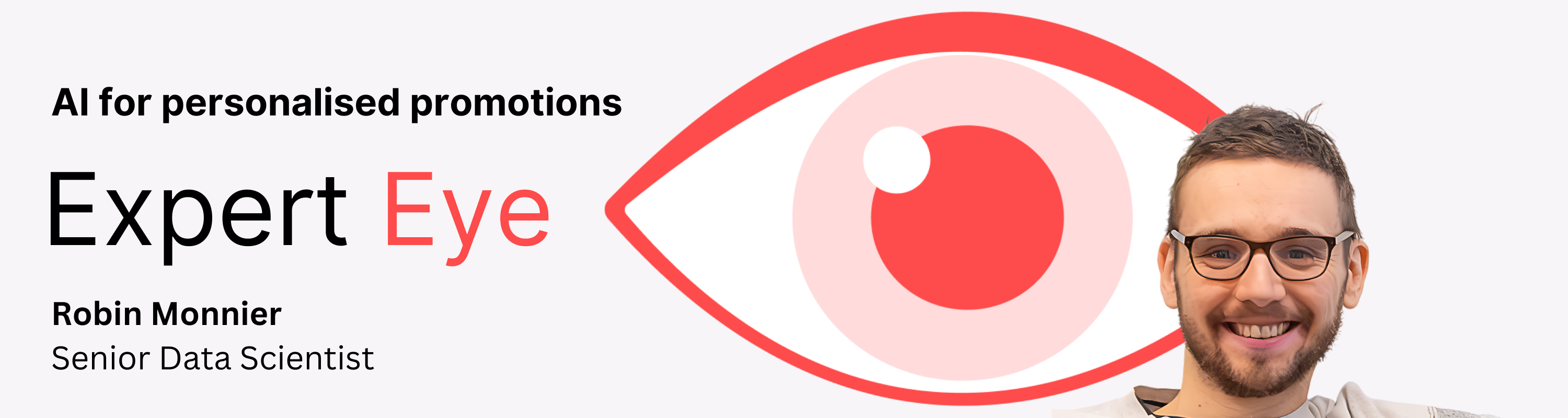
An inside view of Data Science's role at the core of relevanC's personalised promotions platform, Lead Data Scientist, Robin Monnier
5 min read
Traditionally, personalised promotions have been based on broad customer segmentation. How do you ensure more effective targeting and personalisation of promotional offers for both retailers and brands?
We employ the latest advancements in AI to accurately predict customer receptivity to promotional offers using customer purchase history and product sales history. Additionally, we empower our suppliers to refine the scope of targeted customers by our algorithms through the creation of personalized segments. It is by combining these two approaches, business expertise and AI, that we are able to deliver the best offers to customers while meeting the marketing objectives of suppliers (loyalty of existing customers, recruitment of new customers or reactivation of lapsed customers).
What technical challenges did you encounter in developing a model to combine customer receptivity prediction with brands' personalised segments, and how did you overcome them?
Personalized promotion poses AI challenges not necessarily found in other applications, as it is difficult to evaluate the performance of models before they are deployed in production. If a new version of the model predicts that a customer is receptive to an offer from a past campaign, we cannot know if the customer would have actually used it as that customer did not actually receive the coupon!
In this context, we need to measure the impact of a model on real campaigns by deploying it in production as soon as possible. This is why we have developed tools that allow us to use multiple algorithms in parallel (or multiple versions of the same algorithm) and compare their performance in real time. This also allows us to gradually deploy a model, allocating an increasing proportion of customers to it, and thus minimise risks for both suppliers and retailers.
This new update also allows each offer to be assigned a specific marketing objective. Can you explain how the model adapts its algorithm based on the objective (for example, maximising sales or reactivating lapsed buyers of the brand)?
Each brand defines its own marketing objectives, such as recruiting new customers or retaining existing buyers. For example, a brand with the objective to attract new customers may prefer to target shoppers who purchase products from complementary ranges to the products in their offer or target customers who purchase similar products from competing brands. This is why we have chosen to let the supplier create a personalised customer segment for their offer, relevant to their own marketing criteria, using an advanced segmentation tool.
Our algorithm will then select from a pool of shoppers in the brand’s personalized segment, choosing the most receptive by analysing a wide range of variables calculated from the customer's purchase history and product sales. These variables provide a complete description of the customer's purchasing behaviour, both for the products in the offer and for similar products from other brands, allowing the algorithm to adapt to different marketing objectives.
How does the system handle situations where customer data for specific products is limited? Are there specific techniques for dealing with data scarcity?
We work with brands whose objective is to promote new products or seasonal products that are sold for a short period each year. For this type of product, we have access to very little purchase history and, therefore, little data to train our algorithms. In this case, we can estimate a customer's receptivity by analysing their purchases of similar products, products in the same category or products of the same brand. In addition, for new customers of the retailer, who have made few purchases, we can determine customers with a similar profile using Collaborative Filtering techniques commonly used in recommendation systems. This means our algorithms remain effective even when promoting new products or new customers, even if, of course, the predictions get more accurate with more data.
What evaluation measures are used to assess the effectiveness of the product segmentation and AI matching approach? Are there specific techniques for model interpretability?
To evaluate the model's performance, we measure its Average Precision, which is a standard metric in Machine Learning in regression problems. From a business perspective, this means comparing the utilisation rate of customers on different levels of receptivity scores predicted by the model. Concretely, a high performing model should have a very high utilisation rate on the top 100 customers (whose predicted receptivity score is the highest), slightly lower on the top 1000, even lower on the top 10000, and so on. This allows us to ensure that the model has a good understanding of customers and offers. We’re seeing a utilisation rate of over 90% on the top 100 most receptive customers, which testifies to the reliability of the model!
Moving forward, what are the possible development paths for the AI model? Are there any specific algorithms or techniques that you would like to explore for future updates?
We are extremely pleased with the performance of our skill score prediction model, to-date. But that doesn’t mean we can’t do better. We are exploring several avenues for development: optimising budget allocation between two competing promotions, using the algorithm to help brands improve the attractivity of their promotions, and recommending optimal budget and date levels for each promotion - this feature is already live on the platform, and we have been continuously improving it since its launch earlier this year.
This approach reflects our commitment to always providing an optimal promotional experience for our customers and users, the group's brands, and their suppliers. We also recently organized an internal hackathon bringing together all of RelevanC's data scientists to generate new ideas, some of which have shown very promising results!
Leave a Comment